FEEDBACK: AISC Proposal w/ Remmelt Ellen
Download MP3In this episode I discuss my initial research proposal for the 2024 Winter AI Safety Camp with one of the individuals who helps facilitate the program, Remmelt Ellen.
The proposal is titled The Effect of Machine Learning on Bioengineered Pandemic Risk. A doc-capsule of the proposal at the time of this recording can be found at this link.
Links to all articles/papers which are mentioned throughout the episode can be found below, in order of their appearance. MegaSyn: Integrating Generative Molecule Design, Automated Analog Designer and Synthetic Viability PredictionDual use of artificial-intelligence-powered drug discoveryArtificial intelligence and biological misuse: Differentiating risks of language models and biological design toolsModel Organisms of Misalignment: The Case for a New Pillar of Alignment ResearchShadow Alignment: The Ease of Subverting Safely-Aligned Language ModelsFine-tuning Aligned Language Models Compromises Safety, Even When Users Do Not Intend To!unRLHF - Efficiently undoing LLM safeguards
In this episode I discuss my initial research proposal for the 2024 Winter AI Safety Camp with one of the individuals who helps facilitate the program, Remmelt Ellen.
The proposal is titled The Effect of Machine Learning on Bioengineered Pandemic Risk. A doc-capsule of the proposal at the time of this recording can be found at this link.
Links to all articles/papers which are mentioned throughout the episode can be found below, in order of their appearance.
- MegaSyn: Integrating Generative Molecule Design, Automated Analog Designer and Synthetic Viability Prediction
- Dual use of artificial-intelligence-powered drug discovery
- Artificial intelligence and biological misuse: Differentiating risks of language models and biological design tools
- Model Organisms of Misalignment: The Case for a New Pillar of Alignment Research
- Shadow Alignment: The Ease of Subverting Safely-Aligned Language Models
- Fine-tuning Aligned Language Models Compromises Safety, Even When Users Do Not Intend To!
- unRLHF - Efficiently undoing LLM safeguards
Creators and Guests
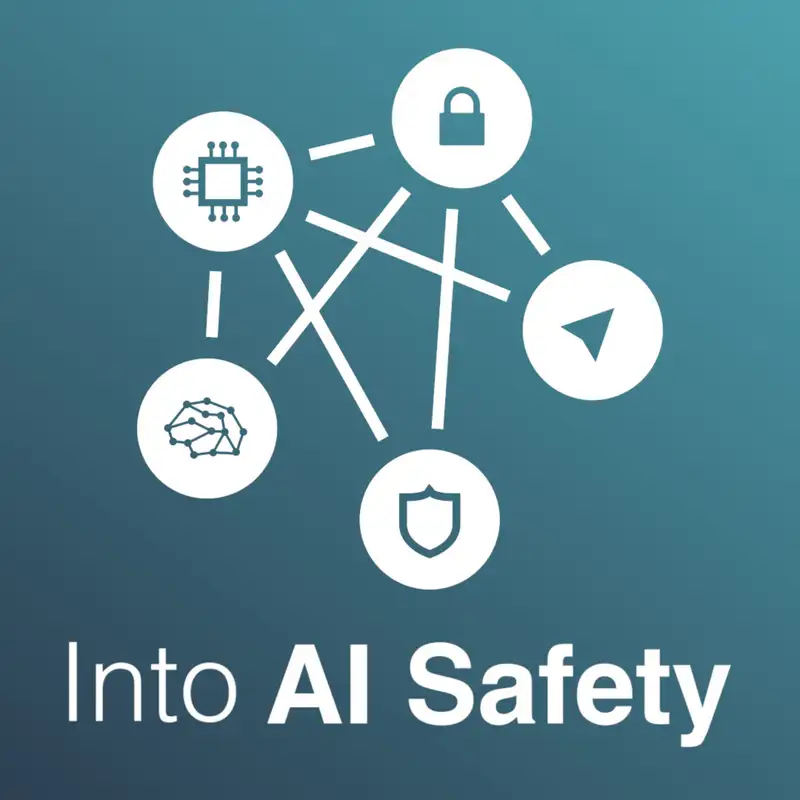